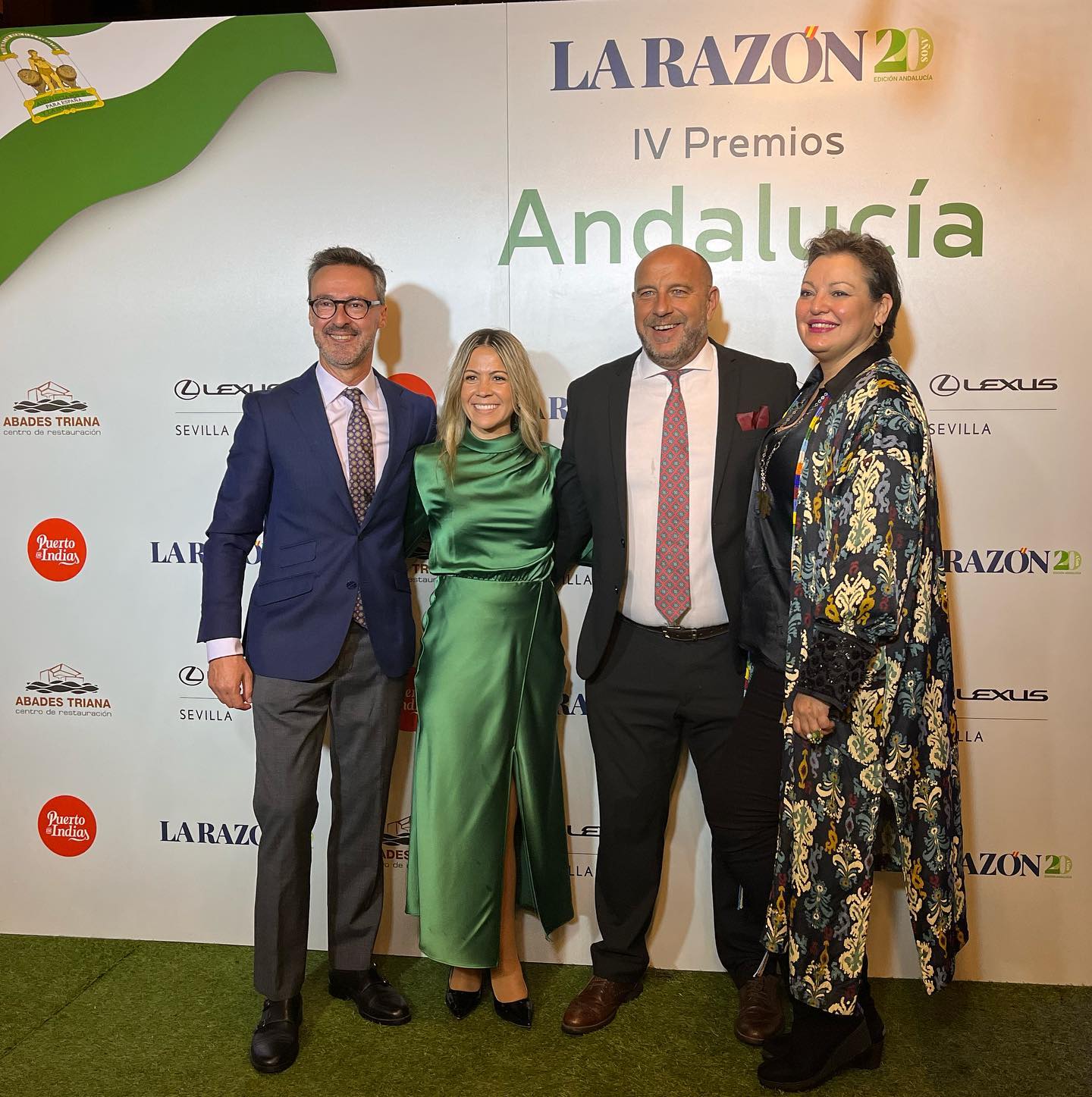
Jean Thompson & Team
Business Partner
The offline deployment of large models on ordinary servers or small intelligent devices has become an irreversible trend. This technological shift is reshaping the underlying logic of AI applications through three key dimensions: hardware innovation, algorithm optimization, and ecosystem reconstruction. Here's a comprehensive analysis based on technological breakthroughs, industrial practices, and policy orientations:
1. Scaled Commercialization of Processing-in-Memory Chips
- Performance Surge: Alibaba DAMO Academy's processing-in-memory chips achieve a 300x improvement in energy efficiency via 3D stacking, embedding computation within memory arrays. Pingxin Technology's N300 chip supports 4/8-bit mixed-precision computing, delivering 0.5 TOPS per core. This enables running 7B-parameter models on standard servers with sub-200ms inference latency.
- Cost Advantages: Leveraging mature 28nm processes, processing-in-memory chips cost 1/5 of GPUs and eliminate the need for additional cooling. For example, Zhicun Technology's WTM2101 chip powers real-time object detection in smart cameras with <1W power consumption.
2. Neuromorphic Computing Breakthroughs
- Energy Efficiency Revolution: Intel's Loihi 2 chip, using spiking neural networks (SNNs), achieves 1,000x better energy efficiency than GPUs in image recognition. Its dynamic reconfigurable architecture adapts to tasks like medical imaging analysis and patient monitoring.
- Real-Time Decision Making: Tsinghua University’s neuromorphic chip enables 0.1ms latency in industrial quality inspection, achieving <0.5% false detection rates.
3. Iterative Model Compression
- Dynamic Structural Pruning: CAS researchers propose a hierarchical compression strategy, retaining 16-bit precision for critical layers and 4-bit for others, resulting in <1% accuracy loss in medical image classification. This compresses 70B-parameter models to 8GB for standard server storage.
- Federated Learning Optimization: Huawei Cloud’s federated learning framework uses differential privacy to train cross-device models without data leaving local domains. In financial风控, it achieves <50ms anomaly detection latency with 3% accuracy improvement.
1. Deep Penetration in Industrial Sectors
- Real-Time Quality Inspection: Haier deployes a 7B-parameter model in smart factories, achieving 99.7% defect detection accuracy via edge servers—5x faster than traditional vision systems.
- Predictive Maintenance: Sany Heavy Industry integrates a 13B-parameter model in construction machinery, reducing downtime by 40% and saving over $100M annually through sensor-based failure predictions.
2. Localized Healthcare Deployments
- Medical Imaging Analysis: United Imaging’s uAI platform runs 30B-parameter models on local servers, enabling real-time CT interpretation with 10x faster diagnosis and offline data security.
- Wearable Devices: Huawei Watch GT 4 integrates a 2B-parameter health model, providing 98% accurate real-time health alerts (e.g., heart rate, SpO2) without internet connectivity.
3. Smart Consumer Electronics
- Mobile AI: MediaTek’s Dimensity 9300 chip supports offline operation of 4B-parameter models (e.g., Tongyi Qianwen), enabling sub-1s multi-turn dialogues with <3W power increase.
- Smart Homes: Xiaomi Home Server runs 7B-parameter models, achieving <500ms response for全屋 automation (e.g., "I’m going to sleep" triggers lighting, AC, and curtain adjustments).
1. Government Support
- New Infrastructure: China’s 14th Five-Year Plan prioritizes edge computing and processing-in-memory chips, aiming to build 500 edge nodes covering 90% of industrial enterprises by 2025.
- Data Security Compliance: The EU AI Act mandates local data processing for high-risk sectors (e.g., healthcare, transportation), driving offline deployment as a regulatory necessity.
2. Open-Source Ecosystems & Toolchains
- Framework Optimization: TensorFlow Lite 3.0 compresses models to 1/4 their original size via automatic operator fusion. Lark Edge Computing reduces cloud reliance to 30% through traffic optimization.
- Development Kits: Huawei Cloud ModelArts offers end-to-end automated tools for model compression, quantization, and deployment, cutting development cycles by 60% for 7B-parameter models on standard servers.
3. Industry Standardization
- Performance Benchmarks: CAICT发布《大模型边缘部署性能评估标准》,defining 12 metrics (e.g., latency, power, accuracy) to standardize edge AI development.
- Hardware Certification: ARM’s Edge AI certification program accelerates model optimization for IoT devices, ensuring compatibility across vendors.
1. Energy & Thermal Constraints
- Dynamic Voltage Scaling: DVFS adjusts chip power dynamically (e.g., 1GHz for inference, 2GHz for training).
- Phase Change Materials: Graphene-based thermal management keeps temperatures <45°C during sustained operation.
2. Accuracy-Compression Tradeoffs
- Hierarchical Compression: Critical layers retain high precision (e.g., 16-bit for financial风控), while others use 4-bit quantization with <0.5% accuracy loss.
- Knowledge Distillation: Teacher models (e.g., GPT-4) transfer knowledge to smaller student models (e.g., 7B), preserving performance during compression.
3. Ecosystem Fragmentation
- Unified Toolchains: ONNX Runtime Edge optimizes deployment across hardware platforms, enabling single-codebase compatibility for servers, mobile devices, and industrial terminals.
- Cross-Vendor Collaboration: The Intel-Qualcomm-MediaTek Edge AI Alliance establishes unified interface standards, reducing hardware adaptation complexity.
Timeline | Technical Progress | Typical Applications |
---|---|---|
2025–2026 | 7B models mainstream on standard servers | Industrial质检, smart homes |
2027–2028 | 13B models on edge devices | Autonomous driving, medical imaging |
2029–2030 | 100B+ models locally deployable | Scientific research, quantum computing |
Offline deployment of large models on standard servers and small intelligent devices represents an irreversible trend driven by:
This transformation transcends technical upgrades, redefining computing paradigms. As ordinary servers and small devices gain autonomous decision-making capabilities, human-AI interaction will enter an era of "implicit computing," where devices anticipate needs without explicit commands. This trend will reshape industries from cloud computing to IoT and smart manufacturing, fostering entirely new business ecosystems.
Business Partner
Business Partner
Business Partner
Business Partner
Indian
Indian